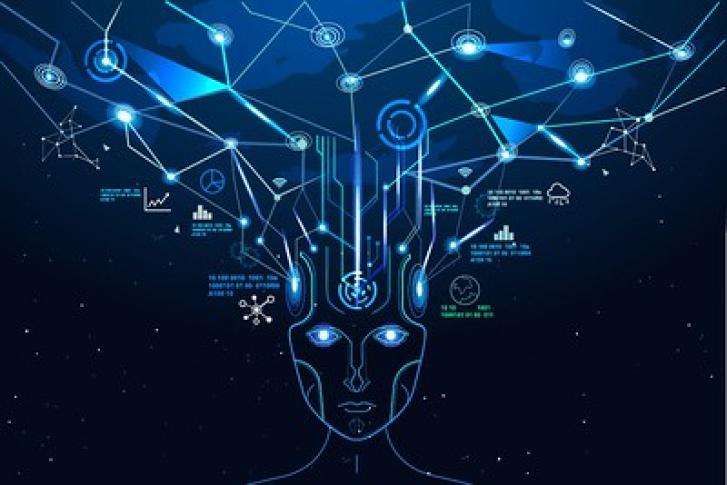
Each year the BBC broadcasts a series of lectures in memory of its founding boss, John Reith. They’re prestigious and intended to be thought-provoking. This year they came from Stuart Russell, who founded the Center for Human-Compatible Artifical Intelligence in California, and were on ‘Living with Artificial Intelligence’.
You can hear or read them here, at least in some countries. This week, some thoughts that they’ve provoked.
Learning to live with change
The idea that we must learn to live with change is hardly new, but we’re hearing more and more of it these days. Governments now tell us, for example, that we “have to learn to live with COVID.”
There are different ways of looking at this, though. On the one hand, fatalism: change is happening and there’s nothing we can do about it. On the other, opportunity: the chance to shape change in ways that suit our other goals.
Fast changes like those that we’re now seeing in technology – like the development of AI – pose greater problems than the slower pace of change that humankind has known to date.
The consequences of fast changes are even more uncertain, more unpredictable and likely to be more diverse in different contexts. We’ve less time to understand them, let alone adjust to them.
And different people have different goals where they’re concerned: winners and losers don’t see the good and bad in change alike, let alone the best ways in which it might be shaped.
There are many questions that arise from this where AI is concerned, that were raised in Russell’s lectures. My focus here’s on three.
How general’s your AI?
A big distinction’s often made between the AI that we have today – which does specific things – and what’s called ‘general purpose AI’: that’s AI that can do whatever, to use a phrase, it puts its mind to; as capable of managing your city’s traffic systems or manipulating public opinion as it is of playing chess.
The proximity of general AI’s dismissed by many AI scientists. We’re a long way from reaching such a thing, they argue, so we needn’t worry much about it. Focus on the here and now, they say, especially the good things in the pipeline, rather than worrying about dystopias that aren’t coming soon.
Russell’s less dismissive. The direction of travel’s clear, he says. The pace of change in new technology’s been faster than predicted, and that will continue. We could reach inflection points – when changes become irreversible – much sooner than we currently expect or hope or fear.
In doing so he’s not alone or new. As long ago as 1951, one of computing’s greatest sages, Alan Turing, predicted that “at some stage, … we should expect the machines to take control”. Stephen Hawking and Elon Musk are among more recent digital celebrities to urge some caution.
Though it’s a long way off, Russell thinks the emergence of general purpose AI would be “the most profound change in human history”. It would at least be “prudent” to prepare for it, and foolish not to think it through till later.
And in the interim?
It’s rash, of course, to juxtapose a present in which AI applications are concerned with specific purposes alone, and an imagined future in which general AI’s prevailed, as if there was no intervening time.
Technology develops over a period of time (if sometimes rather fast and sometimes with sudden leaps). It’s the trajectory of change that matters, each step along the way indicating – and perhaps determining – which next steps follow. Decisions that we make today – to intervene or not to intervene, to regulate or not – set limits on our options down the line.
Those options become more uncertain, too, as our understanding of what technology is doing to or for us becomes less clear to us. This is going to be the case as machine learning takes over more and more of the role that once was played by programmers.
Policies that are concerned with impacts of technology – the opportunities we want to seize, the risks we should avoid – need to focus on two aspects of this. One is the process of transition itself. This should include short- and medium-term implications as well as more distant horizons. The other is the interface between machine and human: where control lies, how decision-making’s done and what objectives govern it.
Exemplifying the short term
In his second lecture, on AI and warfare, Russell gave an example of how the differential rate of change in different sectors affects potential impacts on technology and on society, and how that matters in the here and now.
Weapons systems that can identify, locate and attack targets autonomously, he points out, by which he means without direct human involvement at the time of use, aren’t dependent on general purpose AI and are no longer science fiction. Some are available today, and many more are in development. Mass production would be possible, and so they could be cheap and universal.
We’re closer to autonomous weaponry, perhaps, than to autonomous vehicles. The use or threat of this new weapon generation has implications that reach far beyond geopolitics. The time to regulate them, Russell thinks, is before, not after, those implications have become realities – but he’s alarmed that arms controllers don’t yet see the urgency (and governments would rather have the edge over competitors).
And who decides?
The third question that arises concerns who controls the AI with which we'll soon be living. Here again, the sci-fi imagery associated with AI gets in the way of public policy.
The risks of AI in the public mind rely on images of rogue AI: superintelligent machines with egos, such as Skynet in Terminator movies, that behave like digital Bond villains; or clans of clones, like those in Battlestar Galactica, behaving like colonial powers or genocidal maniacs.
These miss the point. It’s the interaction between human and machine that matters here, not the prospect of the rogue machine. That’s what will determine how opportunities and risks evolve alongside the technology. And how they evolve doesn’t matter only where short-term consequences are concerned. It will also set the frame within which more general purpose AI will develop. (How we regulate today determines how we can regulate tomorrow.)
That relationship between humans and machines is evolving now. Many questions arise and there’s no space here to analyse in depth, but here are some that policy-makers (in technology, in government, business, think tanks, academia and media) should be thinking through.
Five questions at the interface
-
How do AI applications, and the application of AI, alter the relationships between government, business and the citizen? Whose decision-making will prevail where this is concerned (for instance, the impact of analysis of personal data)?
-
What scope is there for common standards – on technology, or impacts such as human rights – including different jurisdictions – in China, Europe and the United States, for instance? Whose standards are most likely to prevail? Come to that, what impact might the United States’ next presidential election have on the possibility of global standards?
-
What will be more important to decision-makers seeking to apply AI in public services: improving the quality of outcomes or cutting costs? And how will decisions on this interact with policies on (in)equality and inclusion? (Think of the tax-cutting priorities of many Northern governments, and revenue deficits of many in the South.)
-
How high’s the risk of failure – through unintended consequences that disrupt or (worse) nullify social and economic policy objectives? Or the risk of accidental errors, or biases in algorithmic choices, undermining confidence in governance and public order? How high’s the risk of malicious or criminal AI, and how containable’s that risk?
-
How do we address the uncertainties we have about what the impact that ‘AI transformation’ will have on society, economy and culture – including things for which we’re unprepared, for some of which we can’t prepare? (Russell considers one of these, employment, in his third lecture, but there are many more.)
Looking ahead
This year’s Reith lectures focus on potential risks of AI rather than on opportunities, but Russell manages a relatively optimistic ending.
AI is coming, he argues, and (in time) that will include the general purpose AI of which pessimists are fearful but that many in the field still say is distant. The question, he suggests, is not concerned with whether machine predominance is coming, but with how we – who have unleashed it – can retain control of the decisions that it makes (and can, it seems, make more efficiently than us). How, in short, do we make it serve us rather than ceding to it our decision-making?
His answer to this is essentially about design: building into AI systems ways of reflecting the diverse needs and goals of different humans (rather than focusing on narrower objectives that may miss important outcomes); building on the experience of standardisation in technology in recent years (not least within the internet).
That’s optimistic because, let’s face it, much current human decision-making hasn’t reached that level of sophistication or inclusion. Including that in the governments and corporations that are heading up AI.
He also thinks that governments – and businesses? – will be prepared to cooperate with one another in order to maximise the benefits and minimise the risks because the advantages of sharing should be greater than not doing so. That seems optimistic too, for two reasons:
-
geopolitical competition, particularly between the global AI powerhouses that are the United States and China; and
-
the disparities in power and influence that exclude most countries in the global South from substantive engagement with the management of new technology.
Big questions, then, without firm answers. Big challenges for which we’re currently not well prepared. Big opportunities, big risks. The important thing to start with, though, is that these big questions are now being asked.
Image: Artificial Intelligence - Resembling Human Brain by deepak pal via Flickr (CC BY-SA 2.0).